Offline Reinforcement Learning Workshop
Neural Information Processing Systems (NeurIPS)
December 12, 2020
@OfflineRL · #OFFLINERL2020
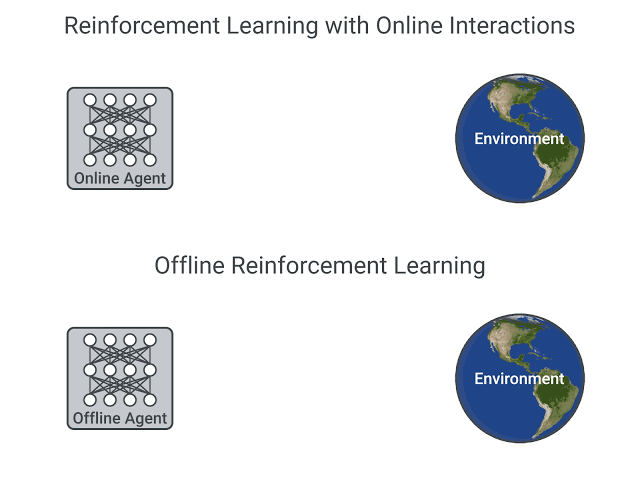
The website for 3rd offline RL workshop at NeurIPS 2022 can be found at offline-rl-neurips.github.io/2022.
The website for 2nd offline RL workshop at NeurIPS 2021 can be found at offline-rl-neurips.github.io/2021.
The remarkable success of deep learning has been driven by the availability of large and diverse datasets such as ImageNet. In contrast, the common paradigm in reinforcement learning (RL) assumes that an agent frequently interacts with the environment and learns using its own collected experience. This mode of operation is prohibitive for many complex real-world problems, where repeatedly collecting diverse data is expensive (e.g., robotics or educational agents) and/or potentially unsafe (e.g., healthcare).
Alternatively, Offline RL focuses on training agents with logged data with no further environment interaction. Offline RL promises to bring forward a data-driven RL paradigm and carries the potential to scale up end-to-end learning approaches to real-world decision making tasks such as robotics, recommendation systems, dialogue generation, autonomous driving, healthcare systems and safety-critical applications. Recently, successful deep RL algorithms have been adapted to the offline RL setting and demonstrated a potential for success in a number of domains, however, significant algorithmic and practical challenges remain to be addressed. Within the past two years, performance on simple benchmarks has rapidly risen, so the community has also started developing standardized benchmarks (RLUnplugged, D4RL) specifically designed to stress-test offline RL algorithms.
Goal of the workshop: Our goal is to bring attention to offline RL, both from within and from outside the RL community (e.g., causal inference, optimization, self-supervised learning), discuss algorithmic challenges that need to be addressed, discuss potential real-world applications, discuss limitations and challenges, and come up with concrete problem statements and evaluation protocols, inspired from real-world applications, for the research community to work on. In particular, we are interested in bringing together researchers and practitioners to discuss questions on theoretical, empirical, and practical aspects of offline RL, including but not limited to,
- Algorithmic decisions and associated challenges in training RL agents offline
- Properties of supervision needed to guarantee the success of offline RL methods
- Relationship with learning under uncertainty: Bayesian inference and causal inference
- Model selection, off-policy evaluation and theoretical limitations
- Relationship and integration with the conventional online RL paradigms
- Evaluation protocols and frameworks and real-world datasets and benchmarks
- Connections to transfer learning, self-supervised learning and generative modelling
Program Committee
- Ajay Mandlekar
- Alex Irpan
- Amy Zhang
- Ankesh Anand
- Aravind Rajeswaran
- Ashvin Nair
- Aurick Zhou
- Avi Singh
- Ben London
- Benjamin Eysenbach
- Bo Dai
- Caglar Gulcehre
- Chen Tessler
- Cosmin Paduraru
- Daniel Seita
- Dibya Ghosh
- Ehsan Mehralian
- Emmanuel Bengio
- Garrett Thomas
- Hadi Nekoei
- Ilya Kostrikov
- Jacob Buckman
- Jae Hyun Lim
- Jiawei Huang
- Justin Fu
- Kamyar Ghassemipour
- Masatoshi Uehara
- Natasha Jaques
- Ofir Nachum
- Ramki Gummadi
- Riashat Islam
- Romain Laroche
- Romina Abachi
- Shangtong Zhang
- Taylor Killian
- Tengyang Xie
- Tianhe Yu
- Xinyang Geng
- Xue Bin Peng
- Yao Liu
- Yevgen Chebotar
- Yi Su
- Ziyu Wang
Organizers
To contact the organizers, please send an email to offline-rl-neurips@google.com.
Thanks to Jessica Hamrick for allowing us to borrow this template.