3rd Offline RL Workshop: Offline RL as a "Launchpad"
Neural Information Processing Systems (NeurIPS)
December 2, 2022
@OfflineRL ยท #OFFLINERL
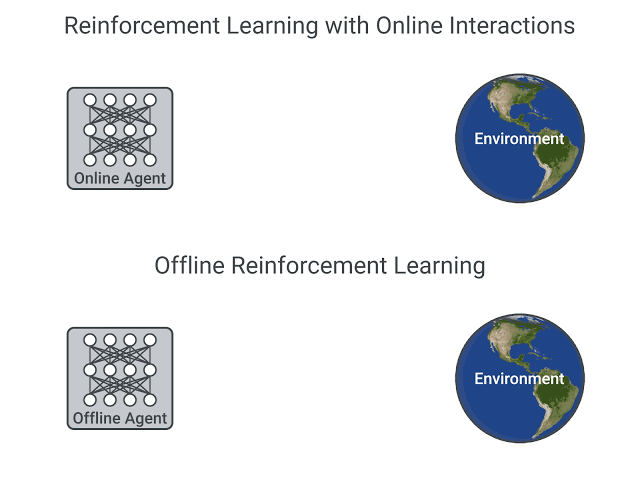
Offline reinforcement learning (RL) is a widely-studied area of study that aims to learn behaviors using only logged data, such as data from previous experiments or human demonstrations, without further environment interaction. It has the potential to make tremendous progress in a number of real-world decision-making problems where active data collection is expensive (e.g., in robotics, drug discovery, dialogue generation, recommendation systems) or unsafe/dangerous (e.g., healthcare, autonomous driving, or education). Such a paradigm promises to resolve a key challenge to bringing reinforcement learning algorithms out of constrained lab settings to the real world.
What's new in this edition? While offline RL focuses on learning solely from fixed datasets, one of the main learning points from the previous edition of offline RL workshop was that large-scale RL applications typically want to use offline RL as part of a bigger system as opposed to being the end-goal in itself. Thus, we propose to shift the focus from algorithm design and offline RL applications to how offline RL can be a launchpad , i.e., a tool or a starting point, for solving challenges in sequential decision-making such as exploration, generalization, transfer, safety, and adaptation. Particularly, we are interested in studying and discussing methods for learning expressive models, policies, skills and value functions from data that can help us make progress towards efficiently tackling these challenges, which are otherwise often intractable.
Topics to be discussed . To this end, we have invited new speakers researching ways to use offline RL as a tool, as well as speakers whose focus areas present new and interesting decision-making challenges which can benefit from using offline RL as a tool. We are also organizing two panel discussions, one focused on discussing decision-making challenges in real-world applications, that can benefit from the offline RL tool, and one focused on understanding the key foundational research challenges in enabling offline RL as a launchpad. We will encourage discussions and contributions around (but not limited to) the following topics that are important in the context of utilizing offline RL as a launchpad:
- Re-using / transferring existing offline RL agents (i.e., reincarnating RL )
- Transfer and generalization of offline RL policies: multi-task and meta-learning
- Pre-training and learning from general-purpose, but unrelated data
- Representation learning for offline RL, and offline RL as a way of representation learning
- Effective methods for fine-tuning offline RL policies, especially to novel problem scenarios
- Fast adaptation and meta-learning of offline RL policies
- Effective exploration via skills learned from offline data
- Sample-efficient online RL via iterated offline RL or deployment-efficient offline RL
- Learning to explore safely using offline data, especially in the presence of other agents
Submission site: https://openreview.net/group?id=NeurIPS.cc/2022/Workshop/Offline_RL
The submission deadline is October 2, 2022 (Anywhere on Earth) September 30, 2022 . Please refer to the submission page for more details.
Organizers
To contact the organizers, please send an email to offline-rl-2022@googlegroups.com.
Thanks to Jessica Hamrick for allowing us to borrow this template.